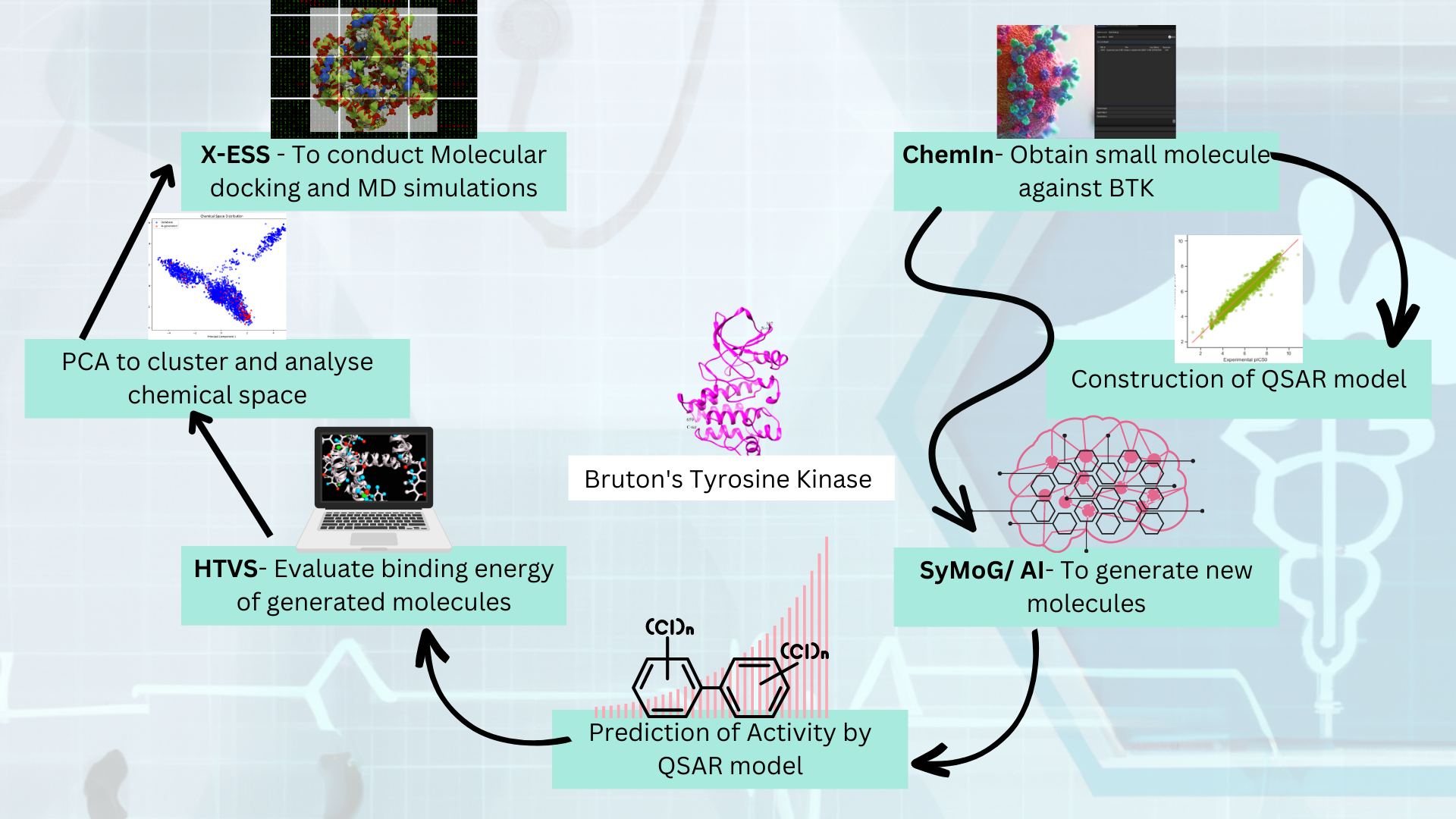
Bruton Tyrosine Kinase (BTK) plays a crucial role in various diseases like Chronic lymphocytic leukemia, Mantle cell lymphoma, Rheumatoid Arthritis, and Psoriatic Arthritis thus making it an attractive target for drug development. However, the effectiveness of current BTK inhibitors is hindered by side effects and the emergence of resistance. To address these challenges, the discovery of novel inhibitors with improved efficacy and safety profiles is essential. In this blog post, we explore an innovative approach that leverages the power of Quantitative Structure-Activity Relationship (QSAR) modeling and Neural Networks to identify promising BTK inhibitors. Specifically, we delve into the methodology employed using the PRinS3 platform software, which revolutionizes the drug discovery process by streamlining data collection, filtering, and molecular generation.
Understanding the Challenges:
Before delving into the methodology, let's first grasp the challenges associated with current BTK inhibitors. While these inhibitors have shown promising treatment in BTK-related diseases, such as certain types of leukemia and lymphoma, without any limitations. One major concern is the occurrence of side effects that can range from mild to severe, impacting patients' quality of life. Additionally, the emergence of resistance poses a significant hurdle in long-term treatment efficacy. To overcome these challenges and improve patient outcomes, the development of novel BTK inhibitors is imperative.
In our quest to discover novel inhibitors against Bruton Tyrosine Kinase (BTK), we employed a comprehensive methodology that harnessed the power of multiple applications within the PRinS3 platform software: ChemIn, SyMoG/AI, HTVS and X-ESS. Let's delve into these applications and their contributions to our study.
1. ChemIn: Streamlining Data Collection and Filtering
We initiated our research by utilizing the ChemIn application within the PRinS3 platform. This application allowed us to access a diverse range of BTK inhibitors from the ChEMBL database and COCONUT database, providing a wealth of data to work with. ChemIn facilitated the seamless extraction of relevant compounds by applying various criteria such as molecular weight, number of hydrogen donors, and acceptors. This meticulous filtering process enabled us to focus on molecules with specific physicochemical properties that were likely to exhibit favorable interactions with the BTK target. Furthermore, the ChemIn application offered the ability to export the selected chemical data in CSV format. This export functionality provided a structured dataset that served as the foundation for constructing a robust 2D/3D Quantitative Structure-Activity Relationship (QSAR) model.
2. SyMoG/AI: Generating Novel Molecules
With a comprehensive dataset of BTK inhibitors at our disposal, we used the SyMoG/AI application within the PRinS3 platform to explore the generation of new molecules. Powered by generative AI, SyMoG/AI employed state-of-the-art algorithms to propose novel chemical structures based on learned patterns from the input dataset. In our study, we subjected the generated molecules to the established QSAR model constructed using the ChemIn application. Remarkably, the majority of these new molecules—up to 90%—were predicted to be active against the BTK target. This high hit rate demonstrates the effectiveness of SyMoG/AI in generating structurally diverse yet potent BTK inhibitors.
3. HTVS: Screening for Promising Candidates
To identify the most promising candidates from the generated molecules, we employed the HTVS (High-Throughput Virtual Screening) application within the PRinS3 platform. HTVS employed efficient virtual screening techniques to evaluate the binding energy of the generated molecules against the BTK target. By assessing the binding affinity, we were able to filter out compounds with lower binding energies, narrowing down our focus to those with the potential for strong interactions and high efficacy.
4. PCA: Exploring Chemical Space
As an additional step, we utilized Principal Component Analysis (PCA) to explore the chemical space of the generated molecules. PCA is a dimensionality reduction technique that allowed us to analyze and visualize the structural variations within the compound set. By reducing the high-dimensional molecular descriptors to a lower-dimensional space, PCA provided insights into the distribution, clustering, and diversity of the generated molecules. This analysis further aided in the identification of unique structural features of the AI-generated molecules and informed our decision-making process for selecting the most diverse and representative candidates for further evaluation.
5. X-ESS: Multi-Target, Multi-Ligand Docking and Molecular Dynamics Simulations
In our pursuit of a deeper understanding of the generated molecules binding mechanisms and stability, we turned to the X-ESS application within the PRinS3 platform. X-ESS facilitated multi-target, multi-ligand docking, allowing us to examine the binding of the generated molecules between BTK mutants and the wild type. This analysis enabled us to identify compounds with differential binding properties, which hold potential for personalized medicine and overcoming resistance.
Moreover, using X-ESS app we performed Molecular Dynamics (MD) simulations—a powerful tool for exploring the dynamic behavior of the generated molecules within the BTK binding site. These simulations provided valuable insights into the stability, flexibility, and overall quality of the compounds, aiding in the identification of promising drug candidates.
Conclusion:
The combined utilization of the ChemIn, SyMoG/AI, HTVS and X-ESS applications within the PRinS3 platform has revolutionized our drug discovery studies targeting Bruton Tyrosine Kinase (BTK). These powerful tools streamlined data collection facilitated the generation of novel molecules, enabled screening and identification of promising candidates, and provided insights into the structural variations and chemical space exploration through PCA.
Through the integration of these applications, we have accelerated the drug discovery process, leveraging the vast potential of BTK inhibitors. The PRinS3 platform has emerged as a pivotal resource, propelling our research forward and holding promise for the development of novel BTK inhibitors with enhanced efficacy and reduced side effects. Our methodology exemplifies the potential of computational techniques in driving innovation and transforming the landscape of drug discovery.