Consequences of Machine Learning on Structural Protein Biology
This event was successfully completed
Event Date:
Friday, March 29, 2024 07:30 PM (IST)
Application Area: Biotechnology
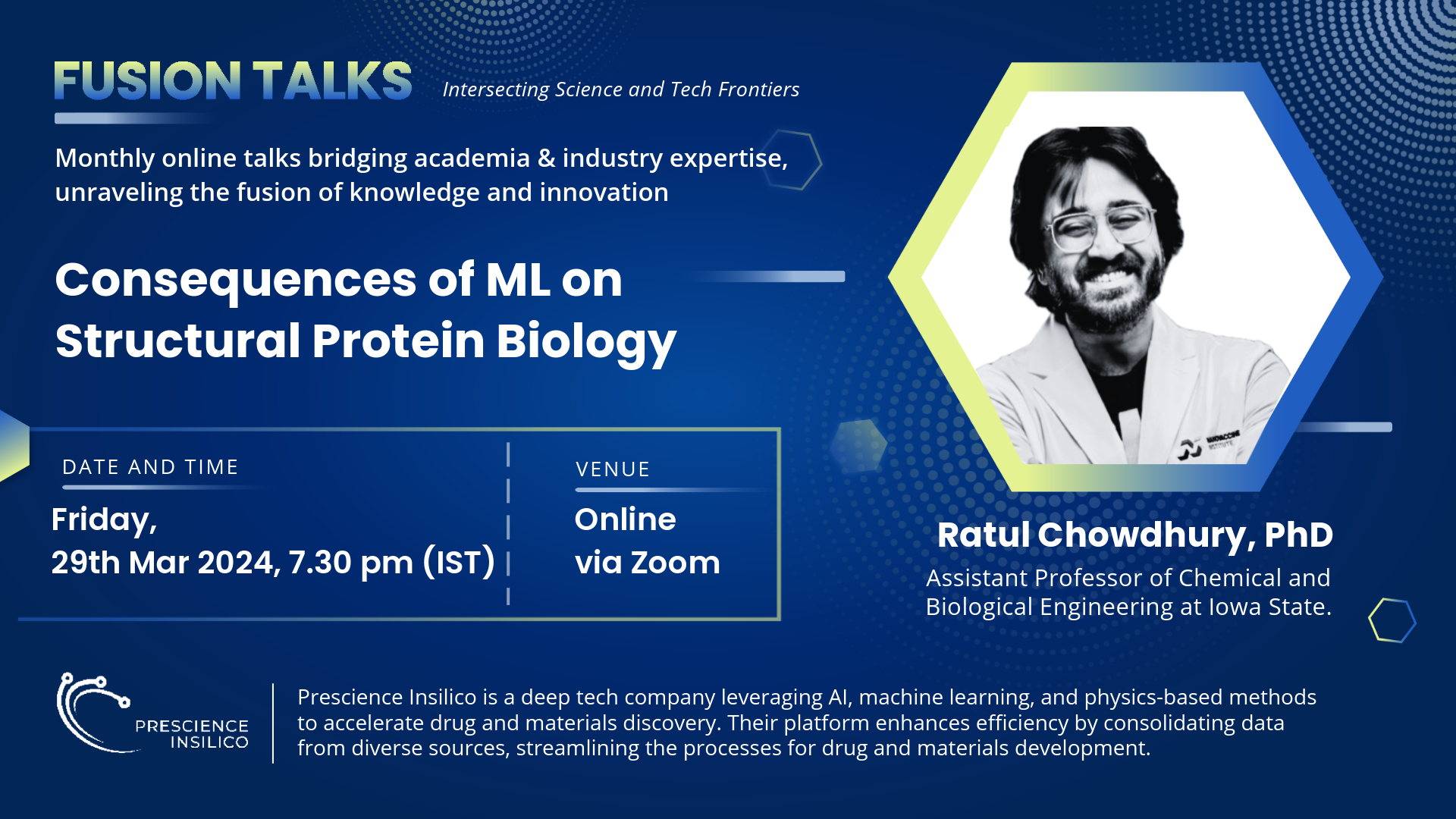
Ratul Chowdhury is an assistant professor of Chemical and Biological Engineering at Iowa State University. Their research group, ChowdhuryLab (Structural Protein Biology and Engineering Lab), focuses on developing and implementing novel machine learning strategies to predict protein structures solely from their amino acid sequences. Their work spans various applications, including drug discovery, biomaterial design, room temperature breakdown of complex molecules, and precise molecular separations.
Prior to joining Iowa State University, Ratul Chowdhury learned their PhD in Chemical Engineering from Penn State University in 2019, under the supervision of Prof. Costas D. Maranas. During his PhD, he developed optimization tools for redesign of (a) channel proteins for separations, (b) enzymes for altered substrate and cofactor specificity, and (c) de novo design of non-immunogenic antibody variable fragments against any disease-causing protein. Next, he was a structural computational structural biology postdoctoral Fellow in the lab of Peter Sorger in the Department of Systems Biology at Harvard Medical School. He devised machine learning strategies to accurately predict the structure of voltage gated ion channels and kinases, and docking a library of drugs to discern non-opioid drug binding modalities with implications in pain and cancer, respectively.
Abstract:
Understanding protein structure involves being able to predict how a small change on an existing protein brings about large functional and structural changes. We are currently laying the groundwork for machine learning models of proteins and their interactions to (a) derive insight about their functions, (b) tune existing proteins for biochemical and pharmaceutical applications, and (c) point-of-care diagnostics. These models extract the ‘grammar’ of protein folding and they have been subsequently used to predict strategies for re-designing existing or de novo protein scaffolds for downstream applications. We focus on predicting protein structure from its amino acid sequence (with and without evolutionary data). Functional annotation maps these protein sequences onto high-dimensional spaces; nearby points are functionally related, where different dimensions correspond to different functional properties. Looking forward, we will also focus on several applications spanning green chemistry, enzyme engineering, protein-pore-based separation devices, and biomimetic tissue design with immunotherapeutic use cases.