SyMoG/AI for Synthetic Molecule Generation, Property Prediction, and Clustering
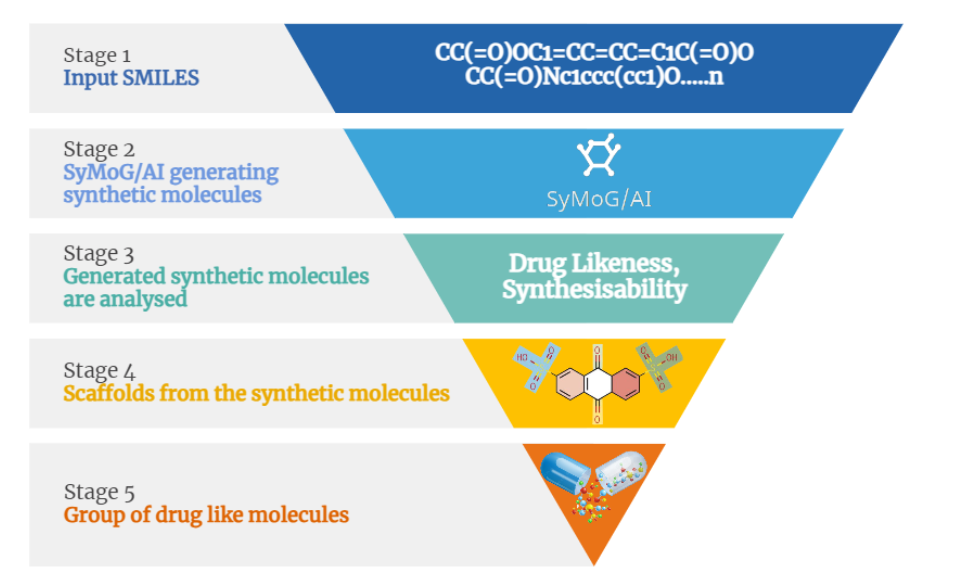
In the fast-paced world of drug discovery, researchers constantly seek innovative solutions to accelerate the process while maintaining accuracy and efficiency. A groundbreaking platform SyMoG/AI in the PRinS3 software suite is making waves by addressing multiple challenges faced by researchers, offering a streamlined approach that spans from the generation of synthetic molecules to the sophisticated technique of molecular clustering. In this blog, we delve into how this platform transforms drug discovery by solving complex issues step by step.
.
1. Expanding the Horizons: Synthetic Molecule Generation
One of the primary issues in drug discovery is the limited availability of suitable drug like compounds for screening. To overcome this hurdle, the SyMoG/AI employs AI-driven techniques to generate synthetic drug like molecules in large numbers. These synthetic compounds not only expand the area of chemical space but also open up new possibilities for drug development. Researchers can construct a dataset of synthetic molecules effortlessly, thanks to predefined generative AI models tailored for various classes of proteins. This eliminates the need to navigate multiple algorithms, providing users with suitable models at their fingertips.
2. Harnessing the Power of AI: Predicting Similar Structures
The SyMoG/AI platform goes further by employing Artificial Intelligence, specifically Graphical Neural Networks (GNN), to predict similar structures based on the input Simplified Molecular Input Line-Entry System (SMILES) notation. This predictive capability simplifies the identification of structurally related compounds, enabling researchers to explore potential candidates for further screening and study.
3. Evaluating Drug Likeness and Synthesizability
In drug discovery, not all molecules are created drug-like. To ensure that generated compounds are viable candidates, the platform offers tools for predicting their drug-likeness and synthesizability. The adherence to established criteria, such as the Lipinski Rule of 5, ensures that only promising molecules move forward in the research pipeline. This feature saves researchers valuable time and resources by focusing on compounds with the greatest potential.
4. Organizing Complexity: Molecular Clustering
Molecular clustering is a critical component of bioinformatics and computational chemistry. It groups molecules into clusters based on chemical properties, facilitating data organization and analysis. In synergy with the platform's capabilities, molecular clustering aids in the selection of diverse compounds from various clusters generated by AI. This process ensures that the training set for Quantitative Structure-Activity Relationship (QSAR) modeling covers a broad chemical space, enhancing the reliability and robustness of predictive models.
In summary, the innovative platform SyMoG/AI in the PRinS3 Software suite discussed here offers a comprehensive solution to the multifaceted challenges encountered in early-stage drug discovery. From the generation of synthetic molecules that broaden the scope of research to the precise evaluation of drug-likeness and synthesizability and finally, to the strategic application of molecular clustering, this platform simplifies and accelerates the drug discovery process. It enables researchers to focus on what matters most—developing effective drugs—while reducing the time and effort spent navigating complex tasks. With AI-driven advancements, the future of drug discovery is brighter than ever. We invite you to peruse our official website at https://www.prescience.in/ for comprehensive information regarding these applications. To gain access to the platform and acquire all the necessary information for an optimal experience, we kindly request you to reach out to us via email at support@prescience.in and get for information from https://prescience.in/prins/