Unveiling New Molecules: Boosting Disease Datasets in a scenario of limited availability of FDA-Approved Drugs using PRinS3 Platform.
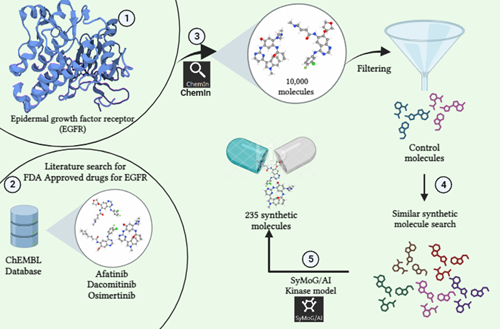
The epidermal growth factor receptor (EGFR) is a key member in a group of receptors responsible for growth, carrying a built-in tyrosine kinase function. In brain tumors, EGFR is often abundant with major internal changes, while lung cancer commonly shows specific mutations and small additions in the kinase section. Due to this, EGFR and its preferred partner HER2/ERBB2 have become prime targets in cancer treatment. When activated by a signal, EGFR triggers a series of timed molecular changes. These include reducing many microRNAs, increasing newly created mRNA, and modifying proteins, collectively controlling genes that determine traits. Besides microRNAs, other types of RNA (long non-coding RNAs and circular RNAs) also play crucial roles in EGFR signaling. EGFR, along with its driving mutations, contributes to metastasis in various ways. By creating communication loops between tumor and supportive cells, EGFR helps in breaking tissue barriers, aiding the survival of groups of circulating cancer cells, and establishing in far-off organs. The different stages of our study is described below.
Here we are starting by generating a dataset of small molecules that could be a possible drug in the future.
Phase 1: The research begins by pinpointing the protein of focus. In this instance, we've selected EGFR (Uniprot ID: P00533) as our target. We then conducted a search within the ChEMBL Database for synthetic compounds associated with this protein.
Phase 2: The second step involves conducting a review of existing literature to identify control molecules related to our target. Through this process, we've identified three specific molecules: Afatinib (CHEMBL1173655), Dacomitinib (CHEMBL2110732), and Osimertinib (CHEMBL3353410) which has been previously studied and FDA Approved for their activity against our target of interest.
Phase 3: Using the ChemIn application within the PRinS3 software suite, we conducted a search for compounds related to our target molecule. This search yielded a collection of 10,000 molecules associated with the target.
Phase 4: We double-checked the output data to ensure accuracy and selected our control molecules based on their ChEMBL ID from the generated results. These chosen controls were then used to search additional structurally similar synthetic molecules, expanding the dataset further.This process aims to find approved or investigational drugs that could potentially work on new targets or diseases. This approach, called drug repurposing or repositioning, speeds up the process by repurposing existing drugs for new therapeutic uses.
Phase 5: Using the SyMoG/AI application's kinase model in the PRinS3 software suite, we created synthetic molecules linked to the target. Inputting data from ChemIn, we analyzed the resulting molecules for synthesizability, Tanimoto score, and counts of hydrogen bond donors, acceptors, and heavy atoms. This process generated a list of 235 synthetic molecules based on the given input.
As the project draws to a close, we've gathered a substantial pool of 1,016 molecules (235 + 781) ready for target screening. Our goal is to predict how these molecules might interact with the target, potentially prompting specific biological alterations. This potential stems from their structural similarities to established control drugs and their anticipated characteristics assessed through AI analysis. The integration of ChemIn and SyMoG/AI within the PRinS3 software has facilitated a smooth flow of data between applications. This seamless integration has significantly expanded our dataset for target screening, instilling confidence in our findings.